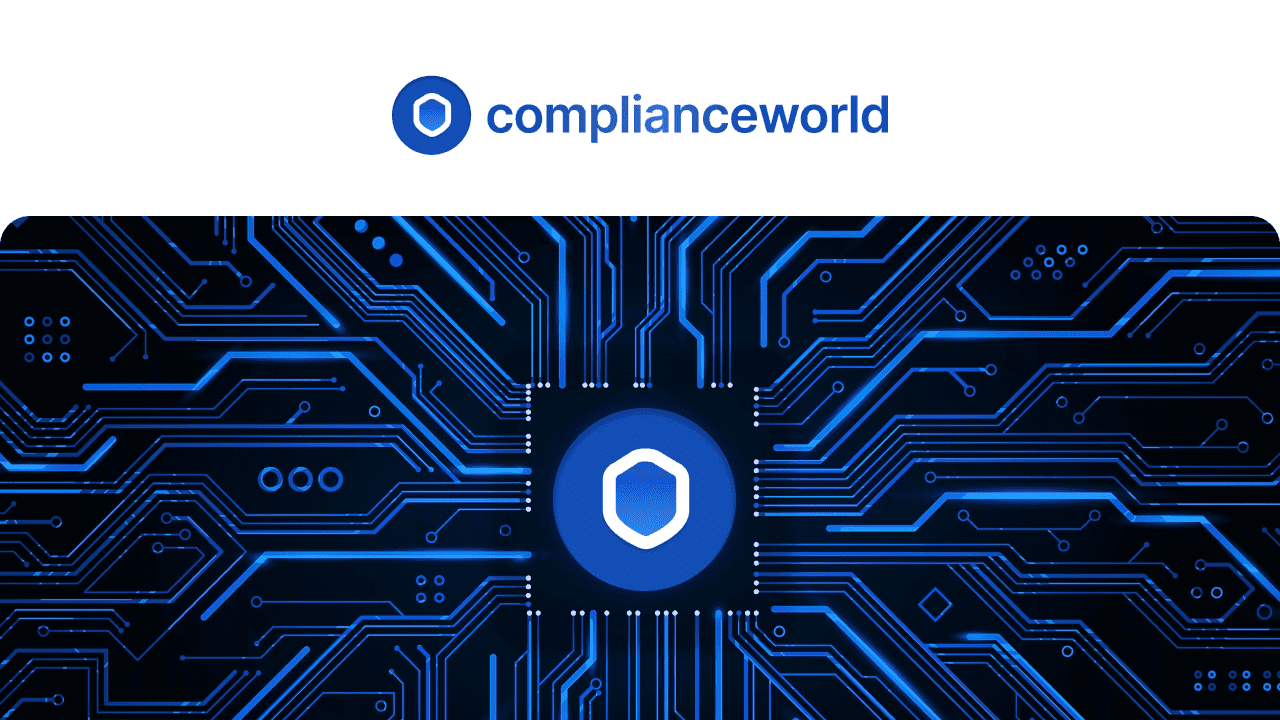
In the digital tapestry of the 21st century, data is the most sought-after commodity. From e-commerce giants to social media platforms, businesses revolve around the acquisition, analysis, and utilization of data. But as the digital footprint of individuals expands, so too do concerns about the sanctity and security of personal information. In this age, data privacy isn't merely a technical term — it’s a fundamental right, a societal concern, and for businesses, a challenge of navigating a maze of regulations. As enterprises grapple with these evolving norms, artificial intelligence emerges not just as a tool but as a compass, guiding the path towards robust digital compliance.
The Data Privacy Landscape: More Than Just GDPR and CCPA
When one broaches the topic of data privacy regulations, the General Data Protection Regulation (GDPR) of the European Union and the California Consumer Privacy Act (CCPA) of the United States often dominate the discourse. And while their introduction marked significant strides in consumer data protection, the narrative of data privacy is more extensive and intricate than these two legislations.
The last few years have witnessed a crescendo of data breaches, from credit agencies to hospitality chains. Each incident has catalyzed a broader societal demand for transparency and an insistence on reclaiming control over personal data. It's a global sentiment that transcends borders. For instance, Brazil's Lei Geral de Proteção de Dados (LGPD) echoes the spirit of GDPR but molds it to fit the unique socio-cultural nuances of its nation. Similarly, India’s Personal Data Protection Bill (PDPB) seeks to strike a balance between individual rights and the booming digital economy, tailoring its regulations to the nation's digital landscape.
Beyond these examples, the Association of Southeast Asian Nations (ASEAN) is witnessing a rapid digital transformation, prompting countries within its umbrella to craft their unique data protection frameworks. Each regulation, though rooted in the universal principle of data privacy, carries its distinct shades, reflecting the socio-economic, cultural, and political contexts of its origin. It's this mosaic of regulations that businesses must navigate, a task both daunting and dynamic.
How AI Interprets and Implements Data Privacy
The global arena of data privacy presents a multifaceted puzzle, one that challenges businesses to decode and adhere to myriad regulations. Traditional methods, constrained by the sheer volume and complexity of these laws, often lag in real-time compliance. This is where the prowess of AI enters the scene.
Harnessing the capabilities of Natural Language Processing (NLP), AI can digest vast troves of legal documents, dissecting the often dense jargon of regulatory texts. This automatic interpretation translates into actionable insights for enterprises, ensuring that even the subtlest nuances of laws don't go unnoticed. But AI's involvement doesn't halt at interpretation.
As businesses hoard exponential amounts of data, pinpointing and categorizing sensitive information becomes a herculean task. AI tools adeptly comb through these vast digital repositories, tagging and classifying data. This automated data discovery ensures that personal or sensitive information is not just identified but treated in accordance with global data protection standards.
Beyond identification, AI's predictive analysis capabilities act as a guardian of digital fortresses. By recognizing irregular patterns or potential vulnerabilities, AI provides an early warning system against potential data breaches, enabling companies to fortify their defenses proactively.
Empowering Consumers through AI
The narrative of data privacy is incomplete without acknowledging the core stakeholder: the consumer. As regulations empower individuals with rights over their data, businesses are pressed to respond efficiently to these entitlements.
Imagine a user seeking to understand their data trail on a platform. Instead of wading through layers of customer support or clunky interfaces, AI-driven privacy dashboards offer a seamless experience. They provide users with lucid, comprehensive insights into their data's journey, fostering a climate of transparency.
Further, as consumers globally exercise their right to data access or portability, AI automates these intricate processes. Be it generating a comprehensive data report for a user or facilitating data transfer between platforms, AI ensures that businesses not only meet these requests swiftly but also in a manner that's compliant with respective regulations.
Moreover, the vision of AI in data privacy extends to educating the masses. AI chatbots and virtual assistants, equipped with the knowledge of privacy rights and regulations, can guide users through their data-related queries, making the concept of data rights accessible and understandable for all.
Can AI Truly Be Privacy-Compliant?
The fusion of AI with data privacy compliance represents a paradoxical challenge. On one hand, artificial intelligence promises to revolutionize the way businesses understand and adhere to data privacy laws, becoming an indispensable ally. On the other, the inherent nature of AI—especially machine learning models—demands vast amounts of data for training, a requirement that can seemingly clash with the principles of data minimization and purpose limitation enshrined in many privacy regulations.
The apprehension stems from legitimate concerns. AI models, particularly deep learning networks, are often described as 'black boxes.' The opacity of their decision-making processes can sometimes make it challenging to ascertain whether the data utilized respects privacy norms. For instance, if an AI model is trained using personal data without proper anonymization, it could inadvertently reproduce or reveal sensitive information, even if the original data is deleted.
However, the realm of AI is not blind to these challenges. Emerging techniques aim to reconcile AI's hunger for data with the imperatives of privacy. Differential privacy, a concept gaining traction, introduces "noise" to the data, making it hard to trace back specific entries to individual users. The result is an AI model that benefits from the data's patterns without compromising the individual's privacy.
Similarly, federated learning is another promising avenue. Instead of centralizing data, which poses risks of breaches or misuse, AI models are trained at the source (like a user's device) and only share model updates, keeping raw data localized.
Furthermore, transparency and explainability are becoming cornerstones of modern AI systems. By making AI's decision-making processes more transparent, businesses can ensure that they align with ethical and privacy standards. Efforts like open-source AI and model explainability tools are strides in this direction, ensuring that AI’s operations can be inspected, understood, and audited.
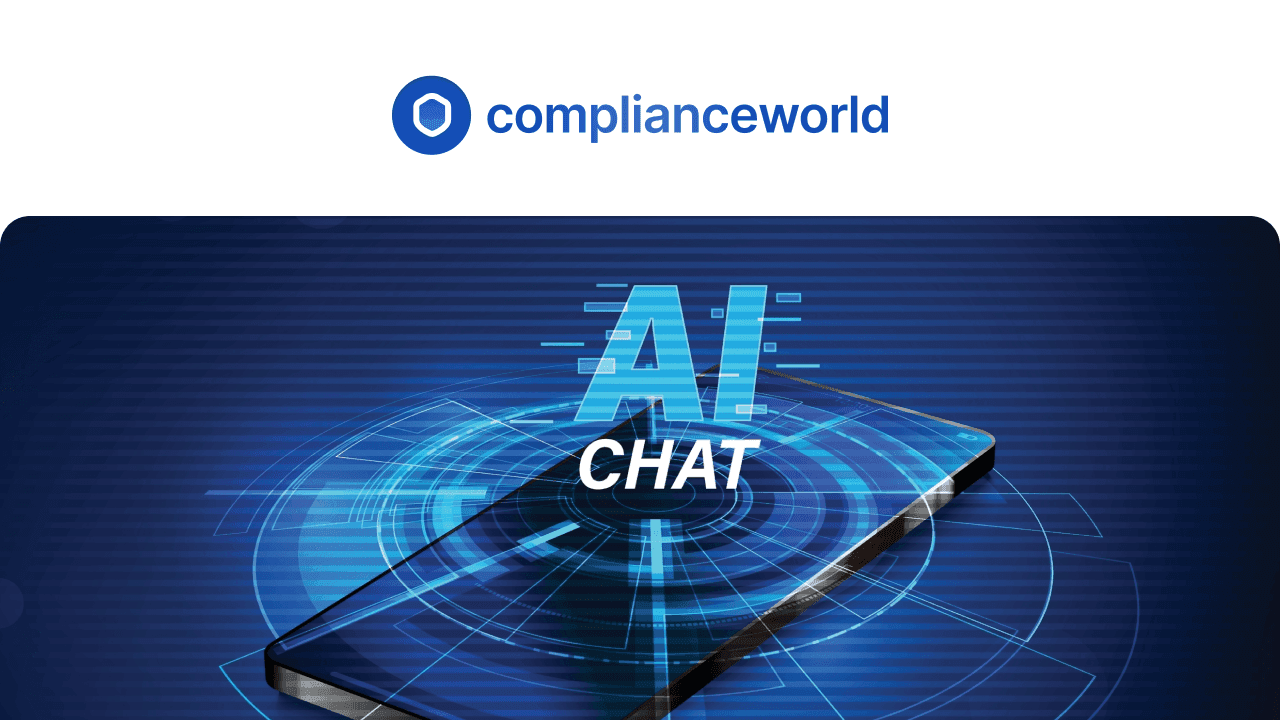
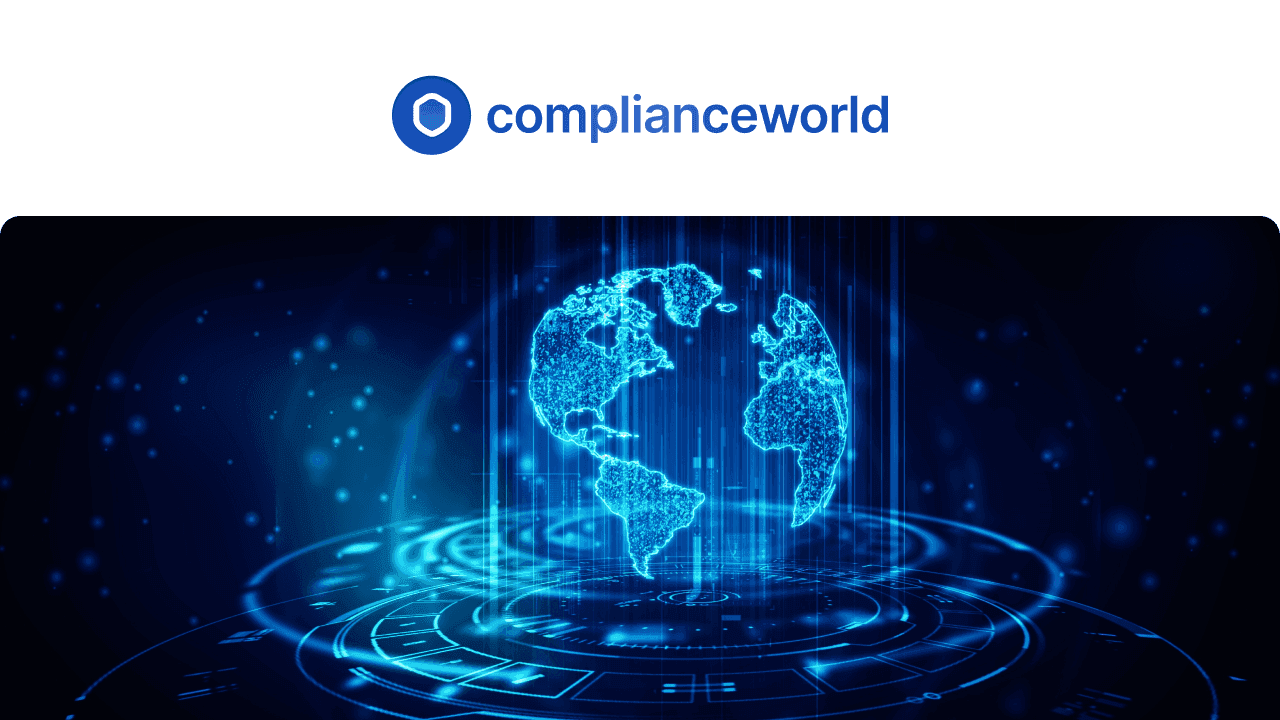
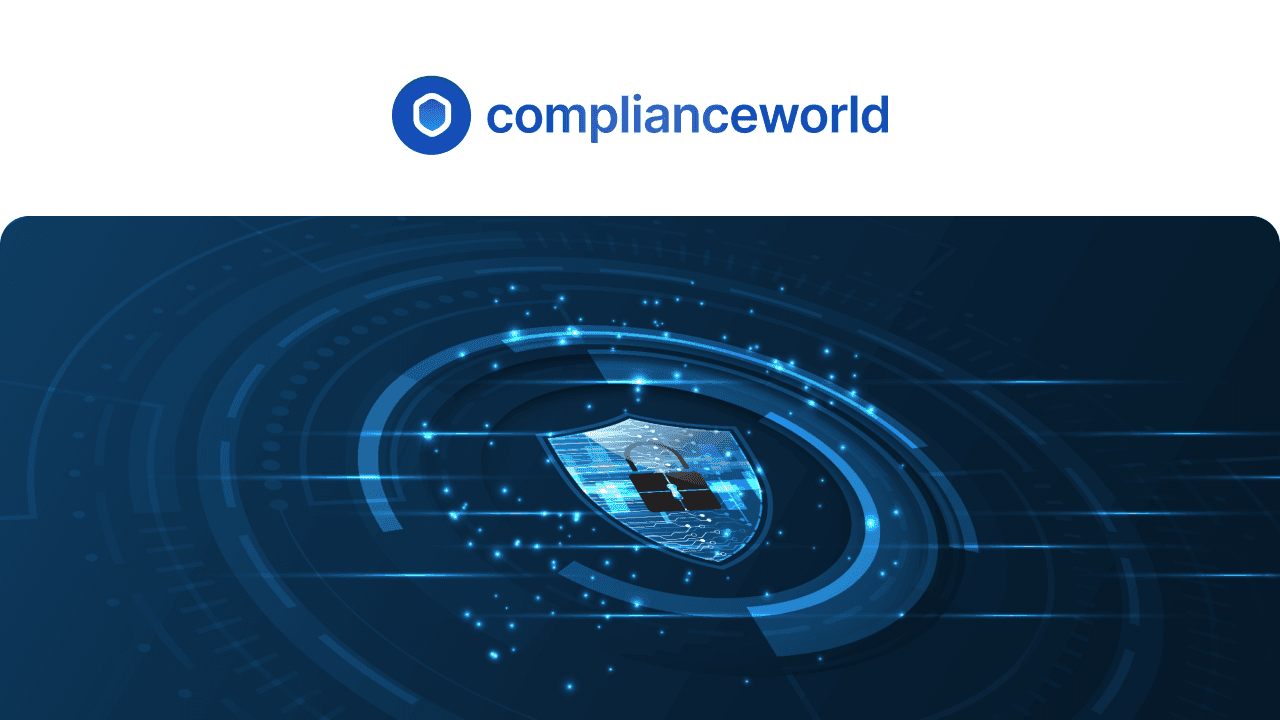
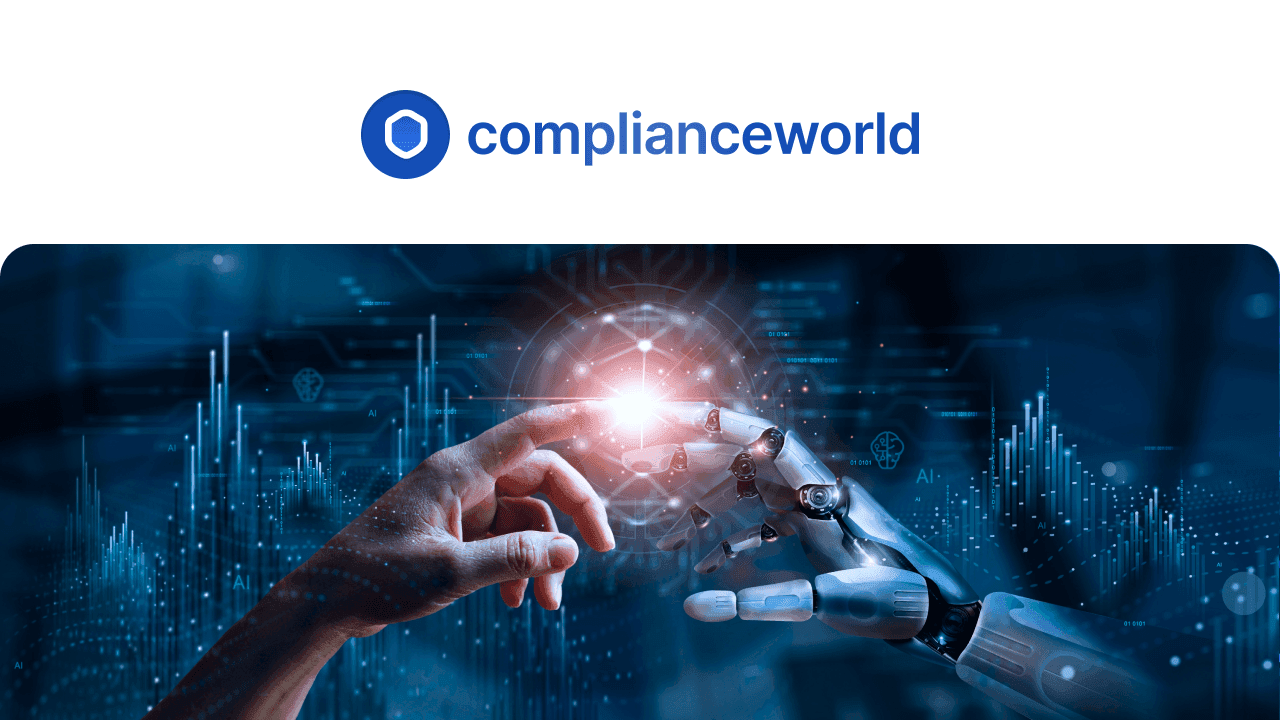
